Machine Learning (ML) based
Advanced Kabaddi Metrics for PKL – Part 1
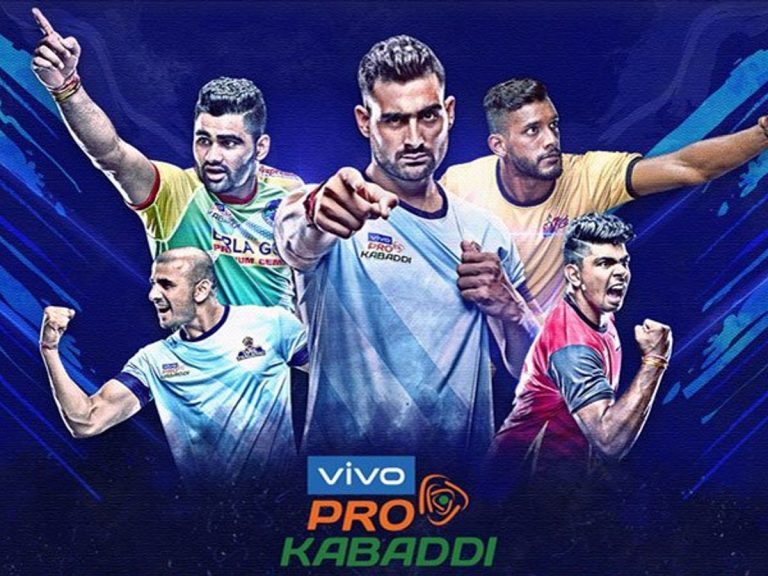
With the advent of the Pro Kabaddi League, the sport of Kabaddi has seen a meteoric rise in its standing in India. While the sport has evolved a lot over these years and its popularity has soared, the tools when it came to measuring performance on the mat, hadn’t witnessed a similar growth over time. The traditional metrics – raid points, tackle points, high 5s and super 10s – don’t always convey the entire picture.
Kabaddi needs a more evolved and a more nuanced system which adds more meaning to the numbers by measuring the net impact they have on the game. Raid Points or Tackle points per game focus on just the points and do not take into consideration any of the circumstances surrounding the raid and that can end up giving an inaccurate representation of how effective a player truly is. With that in mind, we at SportsKPI leveraging Machine Learning models, have come up with a new evaluation metrics that we believe would go a long way towards accurately assessing players, which would help teams in identifying the best of the best in the PKL.
True Raiding Impact (TRI)
We mentioned earlier about the problems with evaluating players with Raid Points per game as the criteria, as it doesn’t take into account the situation in which the player is attempting the raid nor does it take into consideration negative points from failed raids. There are situations within a game where it is easier to rack up points, which may give the impression that a player is performing at an elite level, when actually he is not. To solve this issue, we formed a metric called True Raider Impact (TRI).
Firstly, to deal with the problem with regards to negative points from failed raids, we came up with a metric called Net Point per Raid.
NPpR = (Raider Points scored – Raid Points Conceded) / Total number of raids
NPpR eliminated a fundamental issue with other metrics as it penalised raiders for negative points and it also made it easier to compare raiders at the same level. While this improved the assessment of raiders significantly, it did not consider the situation in which a player went for the raid.
There are many in-game situations or factors that affect the difficulty of the raid. Accordingly, it is important to distinguish between the raiders who are actually good from the raiders who just ‘appear good’ (the ones going for easier raids) by isolating the impact of the context of the game from the raid. The difficulty of a raid was estimated based on a metric called Expected NPpR, which is similar in a way to the Expected Goals (xG) model in football.
True Raiding Impact = Actual NPpR – Expected NPpR
This isolates the match context from the raider’s raids. Raiders who attempt difficult raids will have lower Expected NPpR’s while those who attempt simpler ones will have higher Expected NPpR. This way, the difference levels the platform for all raiders. It now allows us to compare the raiders using the True Raiding Impact (TRI).
TRI revealed that Pardeep Narwal (0.18) was indeed the best raider in PKL 5 contributing an additional 18 point differential for his team when compared with an average raider, followed by Nitin Tomar (0.16), Prashanth Rai (0.15) and Monu Goyat (0.12) while Vineet Kumar (-0.16) brought up the rear end. You can learn more indepth on TRI here
Stay tuned to our social media handles for more advanced metrics
By leveraging ML and AI models in the sport of Kabaddi , we bring value in recruitment and season analysis (Auction, Pre season, Season) for your team, highlighting the importance of each game .For more detailed analysis and potential evidence, please write to info@sportskpi.com
PIC Credit – Prokabaddi.com